The A-Z of Generative AI and ChatGPT – Chapter Q
Every company should be educating its senior executives and board members about Generative AI. It is that important. AI skills are forecast to be the most highly-demanded skills of the next decade, including executives and leaders who understand AI. So, take your productivity, employability, and business to the next level by reading my carefully curated series on Generative AI.
What are others saying about GenAI?
‘Today, there are tools on the market — or freely available on the internet — that allow just about anyone to integrate AI into their workflow.
And the truth is, those who don’t use them are likely to find themselves quickly becoming replaceable. Not because they will be replaced by AI, but because they will be replaced by smarter, more adaptable people who have embraced these tools.
Simply put, those who learn to leverage Generative AI will get more done in a shorter amount of time and create more value for their organizations.’
Source: Boost Your Productivity with Generative AI (hbr.org)
Quality Input to Get Quality Outputs
Crud in, crud out applies more than ever with foundation models. The quality of data input in training models, particularly large language models (LLMs) like ChatGPT, is paramount for ensuring high-quality outputs.
Essentially, these models learn and develop their understanding based on the data they are trained on, adhering to the principle of “garbage in, garbage out.” High-quality, diverse, unbiased, and accurately labelled data ensure that the model generates reliable, unbiased, contextually relevant responses. Conversely, poor-quality data can lead to inaccurate or biased outputs.
What are hallucinations?
One significant issue with subpar data is the risk of “hallucinations” in AI responses. This term refers to instances where an AI model generates information that is either factually incorrect or not grounded in reality.
Hallucinations are often a direct result of training on misleading, false, or low-quality data.
To mitigate this, it’s crucial to:
- Curate and vet training datasets meticulously, ensuring they are representative, comprehensive, and free from misleading information.
- Have more diverse data to avoid biases and enhance the model’s ability to understand and respond appropriately to a wide range of topics and viewpoints.
- Ensure regular updates and refinements to the training data (see supervised learning in Chapter S) to keep the model current with evolving language, knowledge, and societal norms.
In addition, you should also:
- Provide clear and specific prompts that lead to more relevant and accurate outputs from LLMs. Businesses can guide the model toward generating more reliable responses by structuring the input.
- Encourage step-by-step thinking and ask the model to provide facts and references to sources in its responses.
- Link External Data Sources: Incorporate external knowledge sources to verify answers and reduce the likelihood of hallucinations.
- Implement OpenAI’s Reinforcement Learning with Human Feedback (RLHF). This technique involves fine-tuning the model based on feedback from human annotators, which helps improve its performance and reduce hallucinations.
- Eliminate inherent biases and use better regularization techniques to prevent overfitting and improve generalization.
- Use scoring systems where human annotators rate the level of hallucination and compare generated content against baselines.
- Adjust the model’s temperature. LLMs have a temperature parameter that controls the randomness of their output. Lowering the temperature can make the responses more deterministic and reduce hallucinations.
In essence, the quality of data input directly influences the effectiveness, reliability, and ethical integrity of large language models. The better the input data, the better the output of the model.
Need help ? Then contact me.
Need help understanding how to avoid hallucinations or implement Generative AI into your business ❓
Then book a FREE 30 minute introductory call so we can discuss your specific business Data Analytics, Artificial Intelligence, or Generative AI needs – click here.
Quantum Computing and AI
Quantum computing and Artificial Intelligence (AI) might seem as distant from each other as London and Singapore, but they are far more related than you might imagine. According to a McKinsey Global Institute report, the potential value created by AI in various sectors could reach $13 trillion by 2030, with quantum computing acting as a catalyst.
How will quantum computing advance AI?
Quantum computing will accelerate the next generation of Artificial Intelligence beyond anything we have seen. Whilst LLMs are made up of billions or even hundreds of billions of data points, quantum computing will enable LLMs to create correlations between trillions of related data points.
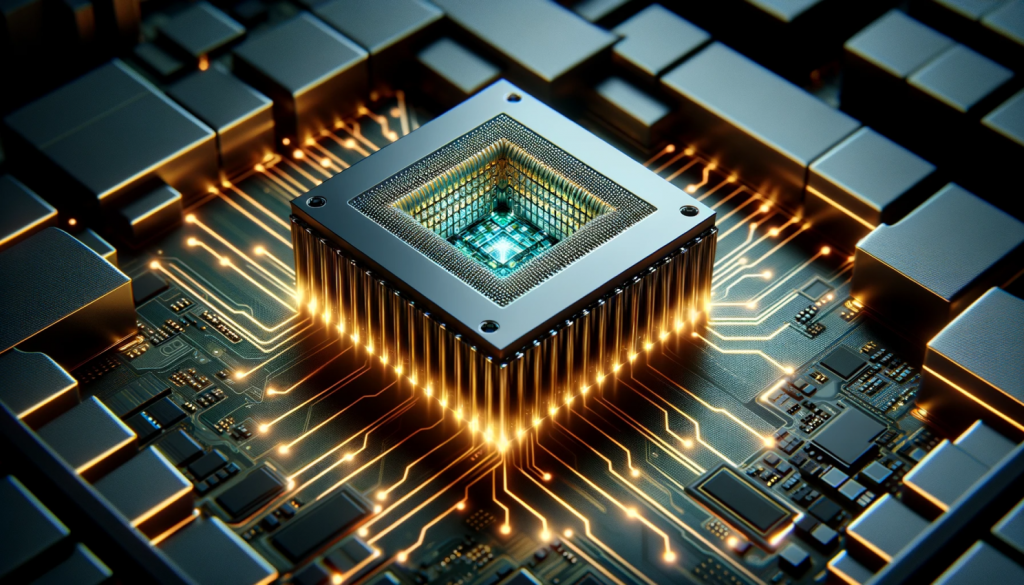
Imagine your regular computer is like a really fast bookkeeper, doing one calculation at a time super quickly. Now, think of a quantum computer as a kind of magical bookkeeper who can do many calculations at once. This magic comes from the quirky ways of quantum mechanics, the rules that govern tiny particles like electrons and photons.
In a regular computer, you have bits that are either 0 or 1, like light switches on or off. But in a quantum computer, you have quantum bits, or qubits. These qubits can be both 0 and 1 simultaneously, thanks to a weird property called superposition. It’s like having a light switch that’s both on and off simultaneously!
Then there’s entanglement, another quantum trick. If you entangle two qubits, changing one can instantly affect the other, no matter how far apart. It’s like having two magical, connected light switches. So, with these qubits being in multiple states at once and instantly influencing each other, quantum computers can solve complex problems much faster than regular computers.
Quantum computing promises major breakthroughs in machine learning and natural language processing. When we throw mountains of data and a lot of models and computational power at a problem, we can solve many really difficult issues.
The synergy between quantum computing and AI would not offer an incremental improvement in LLMs; it would be a paradigm shift, unlocking unprecedented computational capabilities and efficiency. As such, quantum computers could be a game-changer for drug discovery, encryption, trading strategies, portfolio optimization, asset pricing, risk management, retail, e-commerce, genomic analysis, and supply chain optimization!
Exciting times!
Sample prompt to help you understand quantum computing: please act as a Quantum Computing Specialist, delving into the principles and potential applications of quantum computing. Explain the key differences between classical and quantum computing, focusing on qubits, superposition, and entanglement. Discuss current research and advancements in the field and the potential impact of quantum computing on cryptography, optimization, and Artificial IIntelligence. As a specialist, provide a thorough yet accessible overview of this cutting-edge technology and its implications for the future.
Quantum and AI
The convergence of quantum computing, AI, and generative AI technologies is not just an extension of previous AI generations. It represents a fundamental evolution, promising to bring novel solutions and efficiencies across industries.
Keen to learn more❓ Then keep reading.
Letter Q
Understanding the A-Z of Generative AI opens up a rich world of possibilities for business leaders. These concepts provide valuable insights into how AI can create new content, solve problems, and drive innovation across various industries.
1. Quantum Generative AI 🆀
Quantum Generative AI is an emerging field that combines quantum computing principles with generative AI techniques. Quantum computers can perform complex computations and simulations at speeds unattainable by classical computers. Quantum Generative AI explores the potential of quantum algorithms to generate content with unprecedented efficiency and accuracy.
For Example: Researchers experiment with quantum generative models to create new drug compounds more effectively by simulating molecular interactions at the quantum level.
Prompt: “Develop a comprehensive business presentation on ‘Quantum Generative AI.’ Begin with an introduction slide that defines Quantum Generative AI, emphasizing its significance as a merger of quantum computing and generative AI techniques. Include a slide on the advantages of quantum computing, such as its ability to perform complex computations at unprecedented speeds.
Next, create a series of slides detailing how Quantum Generative AI can revolutionize various industries, from pharmaceuticals to finance, with specific examples of potential applications. Include a comparison slide contrasting Quantum Generative AI with traditional AI, highlighting improvements in efficiency and accuracy.
Dedicate a section to the challenges and ethical considerations of implementing Quantum Generative AI in business, such as computational requirements and data privacy concerns.
Conclude with a future outlook slide, projecting how Quantum Generative AI could evolve and its potential impact on global markets. Ensure each slide is accompanied by clear, concise bullet points, and consider using infographics and charts for data visualization.”
2. Querying Generative AI Models 🆀
Querying Generative AI Models involves posing specific questions or requests to AI models to obtain targeted outputs. It’s like asking a precise question to get a precise answer. Querying enables users to interact with AI systems effectively, ensuring content generation that meets their requirements.
For Example: An AI-powered customer service chatbot accepts user queries and provides relevant product recommendations based on customer preferences.
Prompt: “ChatGPT, develop the capability to receive and respond to user queries effectively, generating content that aligns accurately with user inputs.”
3. Qualitative Evaluation of Generative AI 🆀
Qualitative Evaluation of Generative AI involves assessing the quality and relevance of the content generated by AI models through human judgment. It’s like having human evaluators review and rate AI-generated outputs. Qualitative evaluation ensures that the content meets high standards of coherence and accuracy.
For Example: A team of human evaluators rates the fluency and relevance of AI-generated text to ensure the language model’s performance.
Prompt: “ChatGPT, undergo qualitative evaluation by human experts to improve your content generation and maintain high-quality responses continually.”
4. Quantitative Evaluation of Generative AI 🆀
Quantitative Evaluation of Generative AI involves measuring the performance of AI models using objective metrics. It’s like grading an exam based on predefined criteria. Quantitative evaluation provides numerical scores to assess the content generation capabilities of AI models.
For Example: An AI image generator is quantitatively evaluated using metrics like Inception Score or Frechet Inception Distance to gauge image quality and diversity.
Prompt: “Prepare an in-depth report on ‘Quantitative Evaluation of Generative AI.’ Start with an introduction that explains the concept of quantitative evaluation in the context of AI, likening it to grading an exam with predetermined criteria.
The first section should outline the importance of objective metrics in assessing AI performance. Follow this with a detailed analysis of various quantitative metrics for evaluating generative AI models, such as accuracy, precision, recall, F1 score, and perplexity. Include case studies or examples of applying these metrics to assess different generative AI models.
Create a comparative analysis section to show how different AI models stack up against these metrics. Discuss the limitations and challenges of relying solely on quantitative evaluation, highlighting the need for qualitative assessment.
Conclude with recommendations for best practices in quantitative evaluation of generative AI, suggesting how these metrics can be effectively utilized to improve AI development. Ensure the report includes charts, graphs, and tables for data representation, and each section should have clear headings and subheadings for easy navigation.”
5. Query Expansion in Generative AI 🆀
Query Expansion in Generative AI involves enriching user queries with additional context or related information to improve content generation accuracy. It’s like providing more context to a search engine to get better results. Query expansion helps AI models understand user intent better and generate relevant content.
For Example: An AI language model expands a short user query like “best laptops” to “best laptops for gaming under $1500” to provide more precise recommendations.
Prompt: “ChatGPT, develop the ability to perform query expansion to enhance your content generation and deliver more contextually appropriate responses.”
6. Quasi-Monte Carlo Sampling in Generative AI 🆀
Quasi-Monte Carlo (QMC) Sampling in Generative AI is a method to generate diverse and representative samples efficiently. It’s like using a more systematic approach to explore a large space of possibilities. Quasi-Monte Carlo sampling improves content diversity and reduces redundancy in AI-generated outputs.
For Example: In the financial industry, QMC methods are useful for estimating value-at-risk (VaR) and other risk measures. They provide more accurate estimates by reducing the variance associated with random sampling. As a result QMC can enhance portfolio optimization by generating more evenly distributed scenarios for asset returns, leading to better risk-adjusted portfolios.
Prompt: “ChatGPT, Given historical risk data for a fixed income portfolio [Link to Portfolio, I want you to estimate the Value at Risk (VaR) and Expected Shortfall (ES) using Quasi-Monte Carlo (QMC) simulations. Collect historical returns data for the fixed income portfolio AND then compute the daily returns from this dataset. Apply PCA to reduce the dimensionality of the data. Extract orthogonal factors that explain the original variables affecting the portfolio value. Construct the entire term structure by linear interpolation from existing spot rates, which will serve as input for the QMC simulations. Then Generate QMC sequences to simulate scenarios for the portfolio. Calculate VaR and ES based on these scenarios. And finally, compare the QMC results with those obtained using standard Monte Carlo (MC) simulations.”
7. Quick Adaptation in Generative AI 🆀
Quick Adaptation in Generative AI involves rapidly adjusting AI models to new tasks or data distributions. It’s like a versatile athlete who can excel in various sports. Quick adaptation enables AI models to learn from minimal data and adapt to dynamic content generation needs.
For Example: An AI chatbot quickly adapts to new product lines and marketing campaigns to generate contextually relevant sales pitches.
Prompt: “ChatGPT, demonstrate your ability for quick adaptation, learning from limited data to generate content effectively for the random set of customer questions presented in the past 24 hours [Link to customer questions and prior best practice answers].”
8. Question Generation by Generative AI
Question Generation by Generative AI is the capability of AI models to produce meaningful and contextually appropriate questions based on input content. It’s like a curious learner seeking more information. Question generation helps AI models engage in interactive conversations and obtain additional context.
For Example: An AI educational platform generates quizzes with relevant questions based on the content of a textbook or essay question.
Prompt: “Design a detailed lesson plan for a session on ‘Question Generation by Generative AI’ aimed at high school students studying computer science. Start with an introduction that explains the concept of generative AI and its ability to produce contextually relevant questions. Include a brief overview of how this mimics a curious learner seeking more information.
Proceed to a section discussing the technical aspects of question generation in AI, such as natural language processing techniques and machine learning algorithms involved. Incorporate an interactive activity where students analyze examples of AI-generated questions, identifying their relevance and contextuality. Follow this with a hands-on exercise where students use a simple AI tool or platform to input content and observe the questions generated by the AI.
Conclude the lesson by discussing the applications of question generation in AI, such as enhancing interactive conversations, chatbots, and educational tools. Ensure the lesson plan includes clear objectives, a list of required materials, estimated timings for each section, and suggestions for assessment or follow-up activities.”
Productivity Prompts
Embracing Generative AI can lead to enhanced creativity, improved decision-making and a competitive edge in the rapidly evolving data-infused digital landscape.
Generative AI will impact every single role you can think of. Understanding how to interact with ChatGPT will soon be an essential key skill.
Below are five roles that begin with the letter Q with an example prompt, each massively boosting role productivity.
Quality Control Inspector. Quality control inspectors examine products and ensure they meet quality standards.
- Prompt: Implement a standardized quality control checklist to streamline inspection processes.
- Prompt: Conduct regular training sessions to update inspectors on new quality standards.
Quantitative Analyst. Quantitative analysts, or “Quants,” analyze and interpret complex financial data.
- Prompt: Build a predictive model for stock price movements using historical data.
- Prompt: Conduct a risk analysis for a portfolio of financial instruments.
Quality Assurance Manager. Quality assurance managers develop and implement quality assurance policies.
- Prompt: Conduct a root cause analysis to address recurring quality issues.
- Prompt: Develop a quality improvement plan based on customer feedback.
Quantity Surveyor. Quantity surveyors manage construction project costs and contracts.
- Prompt: Prepare a detailed cost estimate for a construction project.
- Prompt: Conduct a cost analysis to identify cost-saving opportunities.
QuickBooks Specialist. QuickBooks specialists handle financial records and accounting using QuickBooks software.
- Prompt: Set up automated financial reports in QuickBooks for regular monitoring.
- Prompt: Provide QuickBooks training to employees to enhance their accounting skills.
Generative AI Research Articles
Some of the best Generative AI articles from some of the best sources on the internet.
- How Quantum Techniques Could Bring Generative AI To The Enterprise (forbes.com)
- Could Quantum Give Us the Generative AI We’re Looking For? | Duke Pratt School of Engineering
- The AI–quantum computing mash-up: will it revolutionize science? (nature.com)
- BCG How People Create and Destroy Value with Generative AI
- ERP Today Generative AI for the enterprise – Pascal BORNET and Kieran Gilmurray
- OpenAI Prompt engineering – OpenAI API
- SSRN: Navigating the Jagged Technological Frontier: Field Experimental Evidence of the Effects of AI on Knowledge Worker Productivity and Quality
- BCG AI at Work: What People Are Saying
- BCG Responsible AI is Crucial to Avoid New AI Tech Risks
Artificial Intelligence Services
Need Data Analytics, Artificial Intelligence, Machine Learning or Generative AI Services❓
Book a FREE 30 minute introductory call so my expert team and I can discuss your specific business needs today – click here.
Comment (1)
[…] you an AI fanatic too? Read my Generative AI series here to dive deeper into the […]